We were honored to participate in the Artificial Intelligence panel at the Standards and Performance Metrics for On-Road Autonomous Vehicles workshop hosted by the National Institute of Standards and Technology (NIST). The panel consisted of government, academia, and industry experts, represented by Mississippi State, University of California Irvine, Robotic Research, Toyota Research Institute, Cruise, and Applied Intuition. These key stakeholders discussed artificial intelligence (AI) for on-road autonomous vehicles (AVs) and how to build trust in AV technology—topics that are increasingly important as the industry develops.
The panel shared insights around the need for standardized and interoperable testing metrics, discussed what security and resilience mean for AVs, and debated current testing practices and gaps. The following takeaways summarize the panel’s discussion around standards and test methods for AVs.
NIST Workshop Takeaways
Prioritize testing based on the frequency and severity of scenarios
According to Hussein Mehanna, Head of Robotics at Cruise, it is impractical for AV programs to strive for 100% test coverage. Mehanna said that AV programs cannot practically focus on every single scenario that might occur in the real world and that many of the most severe scenarios are highly improbable. Hence, AV programs should explore a combination of the most frequent and the most severe events.
The panelists also pointed out that AV programs should not look at averages but instead analyze every individual error, especially when operating in a high-complexity environment.
Use an ensemble of approaches to test the long tail
Vijay Patnaik, Head of Product at Applied Intuition, explained different techniques AV programs can utilize to test low-frequency but potentially high-severity scenarios. The first technique is a traditional approach involving hazard analysis methods such as Failure Mode and Effects Analysis (FMEA).
The second technique is virtual testing. With virtual testing, AV programs take a long tail of scenarios not encountered in public road testing and recreate them in simulation—for example, debris on a highway. Programs can then use simulation to test the AV system in these scenarios.
The third technique allows AV programs to test an AV system on private test tracks and recreate collision-avoidance scenarios based on those tests. No individual AV will be able to handle all of these scenarios, and some level of risk will remain. AV programs thus need to carefully analyze which scenarios there are, what risks are associated with them, how to mitigate those risks, and how the AV’s performance compares to what a human could have done if facing the same situation.
Compare the safety of AV systems to the safety of the alternative
Alberto Lacaze, CEO of Robotic Research, questioned whether AV programs should ask whether an AV system is safe or whether they should instead explore whether the system is safer than the alternative.
“There is a threshold of safety, and it serves as a good guideline for what autonomous systems can do; but beyond that, programs need to look at whether or not that system is better than the average human in a comprehensive measure.”
This approach shouldn’t just include the average case but outlier situations as well. Organizations like NIST and discussions among the AV community can help AV programs navigate this complex analysis.
Applied Intuition’s Approach
At Applied Intuition, we believe that continuous, data-driven verification and validation (V&V) is critical to safely developing, testing, and deploying autonomous systems. AV programs can leverage products like Validation Toolset* for requirements and test case management, scalable test generation, and safety case reporting and analytics (Figure 1).
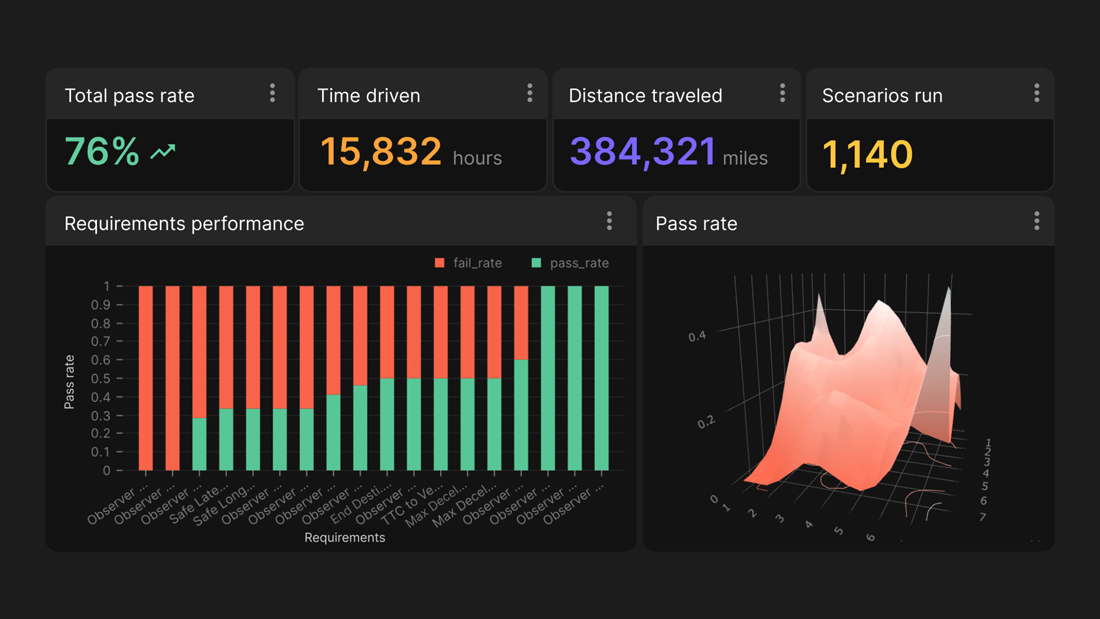
Contact our Validation Toolset team to learn how our products can help mitigate risks and facilitate the development of safe AV systems.
*Note: Validation Toolset was previously known as Basis.