In autonomous vehicle (AV) development, triage—the process of prioritizing and analyzing collected data—is not only crucial for managing the vast volumes of data collected during on-road testing, it is also essential for maintaining system functionality and safety. Triage not only identifies and addresses potential functionality and safety issues, it also manages the growing complexity and scale of AV systems.
This post will explore four key considerations that not only underscore the importance of conducting triage but also guide on how to perform it effectively, focusing on the processes and technological solutions that enhance system performance. We will discuss how strategic data management can accelerate development and improve outcomes, and how Applied Intuition’s integrated tools offer a comprehensive solution for successful triage.
1. Efficient Data Selection
Sensor arrays with multiple cameras and lidars commonly generate terabytes of data each hour. As fleets expand, data volume can reach petabytes weekly. Given the high cost of storing pre-processed data, correctly identifying the most useful subsets of data is crucial for identifying which modules of the AV system need improvement. By focusing on valuable data, data that is not useful can be efficiently deleted or archived, significantly reducing storage and compute costs.
Implementing this approach not only streamlines operations but can also save an AV program substantial amounts in infrastructure expenses. Cumulatively, a large AV program can save millions of dollars on storage and compute infrastructure costs by managing its data efficiently.
Applied Intuition’s data solutions leverage cutting-edge machine learning models to uncover valuable insights from petabytes of collected data automatically, empowering our customers to reduce both time and costs effectively.
2. Streamline Issue Resolution with Tagging and Filtering
Triage not only saves money but also saves time, letting domain experts focus on the most pressing problems. In autonomous vehicle development, specialized engineering groups such as perception, fusion, localization, and planning each play a crucial role. When the AV system encounters issues, it is vital to quickly surface these to the appropriate teams. Advanced tagging and filtering streamline this process by isolating the most critical data points—ensuring that engineers can concentrate on rectifying specific problems aligned with their expertise, thus accelerating improvements in the AV stack.

The significance of data evolves throughout an AV program's lifecycle. In early stages, with fewer vehicles and personnel, almost all collected data may offer valuable insights due to the prevalence of novel scenarios. Here, tagging and filtering are essential for rapidly pinpointing early issues in the stack and channeling relevant data to the right teams.
Early-stage programs, often limited in vehicles and personnel, produce predominantly useful data filled with novel scenarios. Efficient tagging and filtering at this stage quickly highlight critical issues, directing data swiftly to relevant teams.
3. Harness Automation for Efficient Review
The initial challenge in autonomy development involves using data collection vehicles equipped with immature autonomy algorithms, which may not accurately perceive the vehicle's surroundings. Manual inspection of such data is not only time-consuming but also costly.
As programs scale, the explosion in data volume alongside the increasing number of vehicles makes traditional data management methods financially impractical. Notably, much of this data may repeat scenarios already encountered, yet hidden within are rare “long-tail” scenarios critical for testing unexpected conditions.
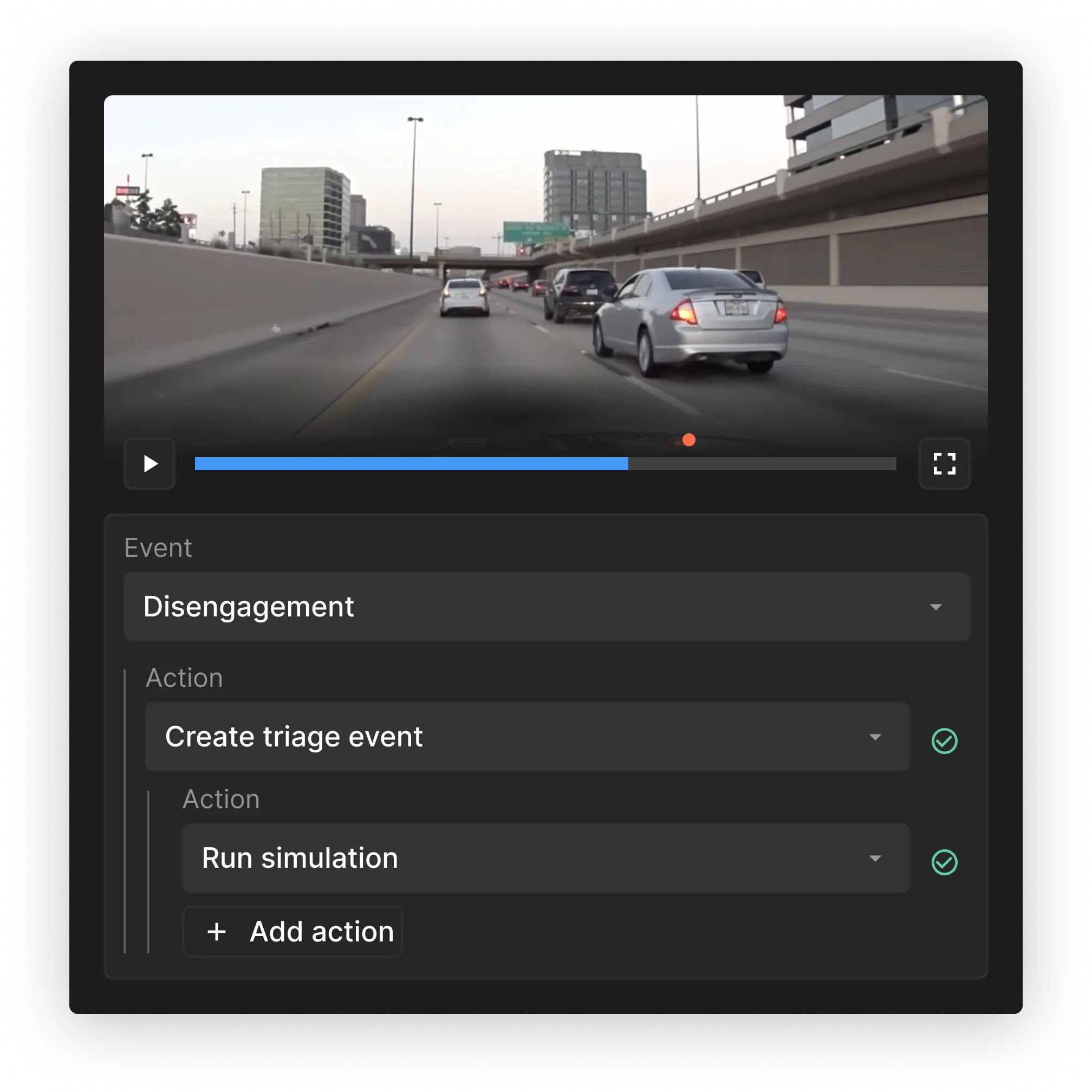
Teams use automation and offline model-generated tags to pinpoint key sections rapidly, allowing engineers to focus on critical reviews more efficiently. Additionally, sophisticated automation and filtering rules allow teams to sift through extensive data volumes efficiently, identifying key areas for further analysis. This layered approach, combining automation with targeted human review, enhances precision in identifying system deficiencies. Instead of poring over data for hours, engineers can spend their time fixing problems most related to their expertise and improving the stack faster.
In the ideal case, real-time event tagging is happening on the vehicle as well. Collected data is being tagged in real-time automatically, and made available to developers. This data could include disengagements and sensor issues, or developers could actually request specific event tags that they can then use immediately in training or analysis.
4. Optimize Data Analysis with Visualization
Visualization tools are indispensable in the triage process, offering a clearer insight into complex datasets and enhancing the decision-making process. By graphically representing data, these tools help engineers quickly identify anomalies and understand critical scenarios, streamlining the review and analysis of vast amounts of AV data.
Engineers use visualization tools to filter logs and identify where autonomous systems disengaged, pinpointing critical issues for targeted problem-solving. With Log Sim, the customer can replay the logged sensor data to the software stack past the point of disengagement and verify if the stack would have entered a hazardous situation.
Additionally, simulation technologies play a pivotal role by allowing teams to extend their analysis beyond initial system disengagements. By replaying sensor data past the point of disengagement, engineers can evaluate whether the system would have coped with ongoing scenarios, thereby assessing the robustness of responses and contributing to enhanced safety measures.
The triage is a core part of the feedback loop to build autonomous vehicle software. With the right tools, teams can improve the value of their data and the efficiency of their triage process. Good tools can help teams analyze the performance of their stack more easily, identify and fix issues faster, find relevant data more effectively, and create better real-world and simulation tests to ensure the quality of their software.
Applied Intuition’s Approach
Applied Intuition’s collaboration with our Level 4 autonomy and automotive original equipment manufacturer (OEM) customers has led to an effective approach to data management and triage.
Data Explorer helps customers tag their data, mine it for insights, and quickly filter their logs to the snippets where their stack became disengaged. In the interest of safety, as a situation becomes risky, the stack will disengage and let a driver take over.
Data Explorer works in concert with other tools like Validation Toolset and Log Sim to help customers develop safer autonomous driving software faster and cheaper.
Contact Applied Intuition to find out more about how Data Explorer, Validation Toolset, and Log Sim combine to develop safer autonomous driving software.